Philipp Schmitt is an artist, designer, researcher and 2019-21 Transformations of the Human fellow at the Berggruen Institute.
When Albert Einstein died in 1955, the pathologist tasked with the autopsy identified the cause of death and then, allegedly on a whim, cut open Einstein’s skull and removed his brain for scientific study. Perhaps he hoped that he’d be able to identify its crucial elements, to be able to hold “intelligence” itself in his hands. The brain turned out to be average in weight, and still to this day we have no neurological insights into its intelligence.
Artificial intelligence researchers follow a different approach to figure out what constitutes intelligence: recreate it, at least in parts. Like Einstein, who was constantly concerned with invisible and abstract matters, the project of artificial intelligence doesn’t lend itself well to our perception. AI does not have a skull to autopsy, which some may consider unfortunate, but it could also be seen as an opportunity to encounter intelligence in isolation. What does it look like?
In European and U.S. popular media, articles about AI and machine learning are commonly illustrated with humanoid characters from classic science fiction movies like “The Terminator,” Ava from “Ex Machina” or Hal 9000 from “2001: A Space Odyssey.” Sci-fi frequently associates the technology with hyper-masculine or hyper-feminine, dystopian or utopian imaginaries and white features (even when the overlord in question doesn’t have a body).
Another theme is circuit boards or binary code collaged with illustrations of brains, often in hues of blue. Here, intelligence is attributed to a mechanistically understood, isolated human brain. Freed from the “burdens” of a body, these images seem to suggest, intelligence reaches its full potential running on bits instead of oxygen.
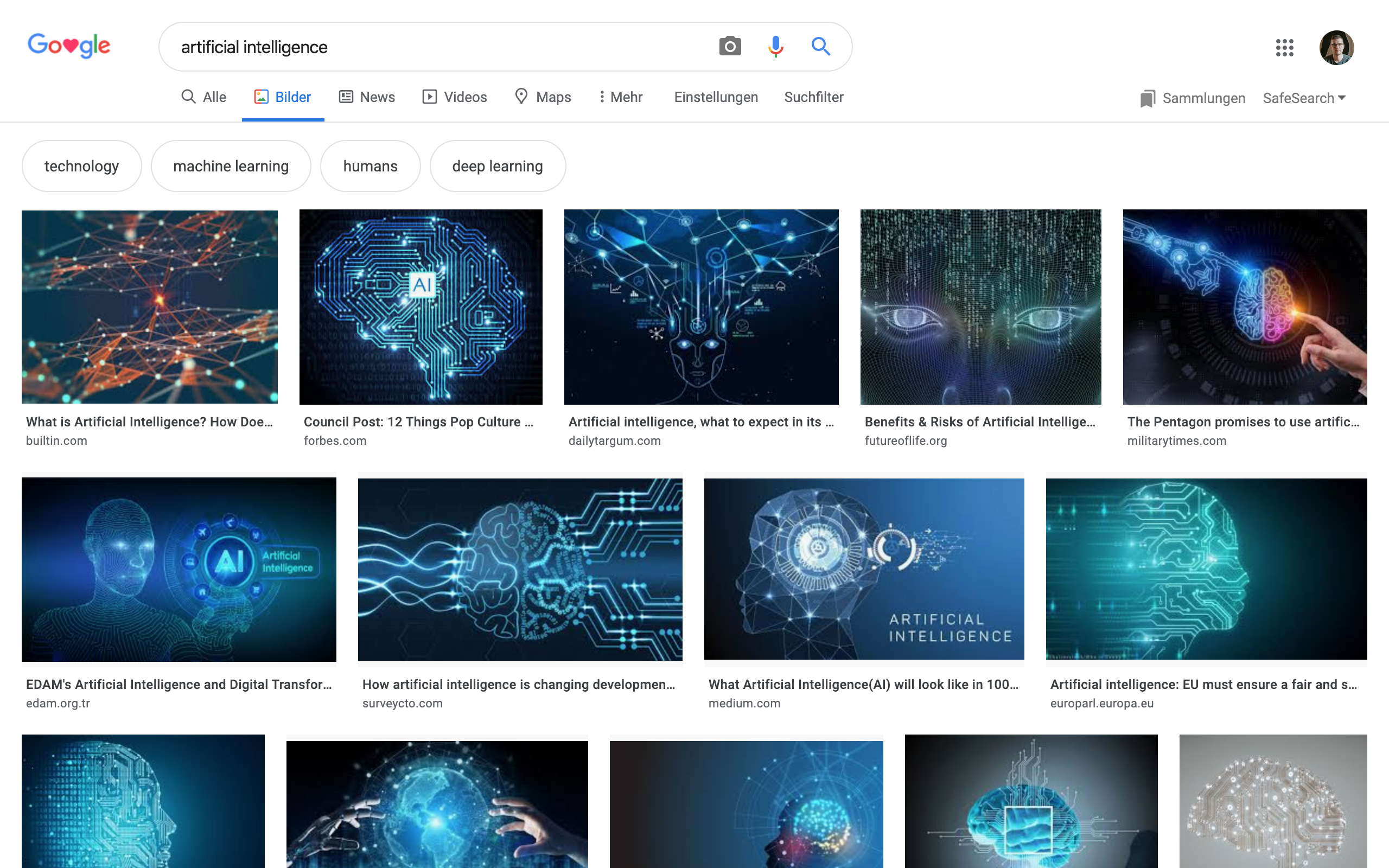
These images are purely decorative and often unrelated to the article. Even worse, they are harmful to the public imagination, because metaphors matter. They influence how we develop, think of and design policy for emerging technologies, just as they have for nuclear power or stem cell research in the past.
While technology narratives can be helpful, popular images of AI taint our understanding and agency. Instead of inviting public discourse around urgent questions like bias in machine learning systems or opportunities to question our understanding of our own intelligence, these images suggest that readers should run from robot overlords or thrust their heads into a blue binary code utopia.
In journal articles, conference posters, textbooks and in the daily life of the New York University lab that I’m a part of, figurative AI imagery is largely absent. Yet there are plenty of images: diagrams of neural networks, figures visualizing equations at work that cannot be witnessed otherwise. These diagrams may seem cryptic to the uninitiated, but to experts they read as architectural blueprints. They depict how neurons are interconnected, how information flows through systems and how it is processed at each stage to produce results.
If there is a picture of contemporary artificial intelligence, I’d argue it is here: in neural network architecture diagrams. I am less concerned with what a diagram might tell a researcher than with the connections between visual representations of neural networks and AI researchers’ conception of cognition. What is at stake with present-day AI is not a robot invasion, but which concepts of intelligence get prioritized, and how they relate to and frame the world at large.
The artificial neuron was invented by the neurophysiologist and cybernetician Warren McCulloch and the logician Walter Pitts. Their 1943 paper introduced an artificial neuron, inspired by the human example.
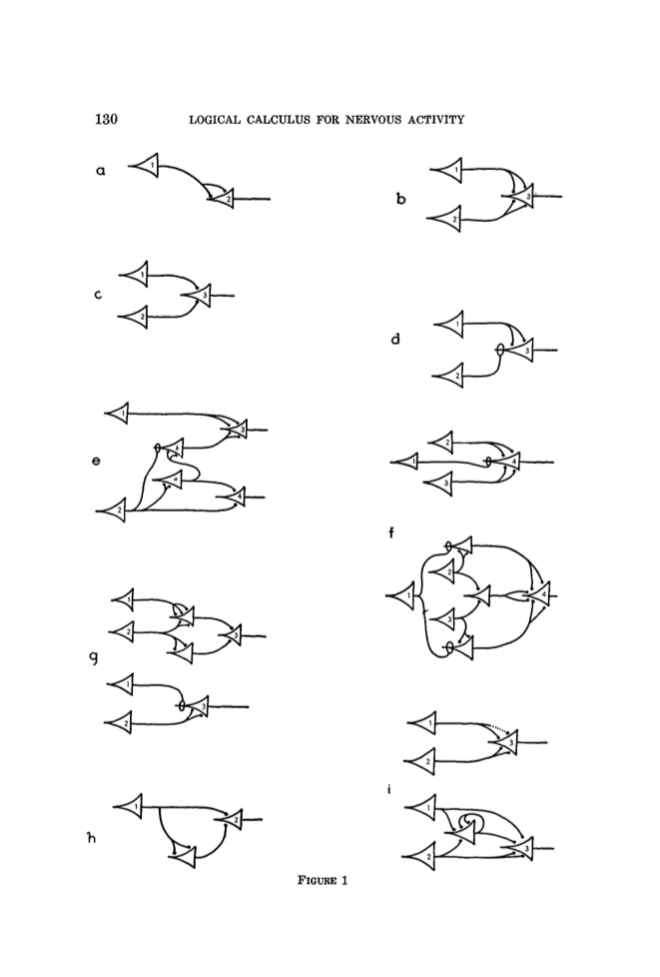
The authors drew neurons by hand as connected, triangular shapes reminiscent of the intricately illustrated drawings of the neural brain by the father of neuroanatomy, Santiago Ramón y Cajal. Abstracted from Cajal’s anatomical rendering towards a modular, diagrammatic representation, McCulloch and Pitts rendered neurons as modular building blocks that can be freely recombined to create neural circuits. As the historian of science and technology Orit Halpern writes in her book “Beautiful Data,” their work rendered “reason, cognitive functioning, those things labeled ‘mental,’ algorithmically derivable from the seemingly basic and mechanical actions of the neurons.”
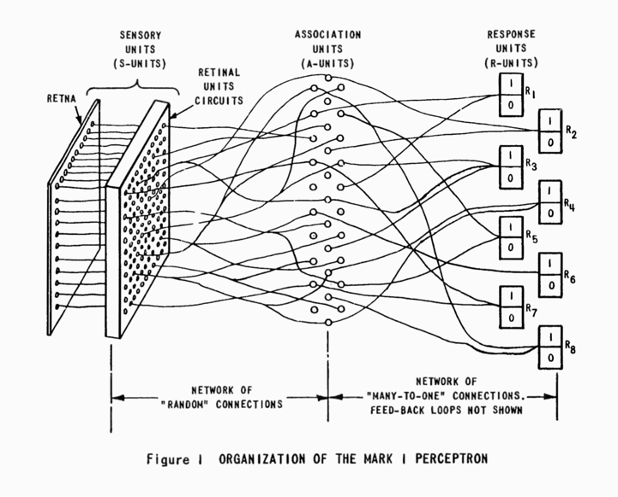
Other early neural network designs also borrow from anatomical concepts and terminology. Frank Rosenblatt’s “Perceptron,” both a simple visual classification algorithm and a room-sized machine, is richly illustrated with beautiful drawings in organic strokes, often reminiscent of cell-like structures. But the perceptron’s “retina” is square, unlike any organism with eyes, and Rosenblatt’s team experimented with different types of diagrams, perhaps illustrating a desire to overcome laborious pseudo-anatomical references to find a different way of representing cognitive functions.
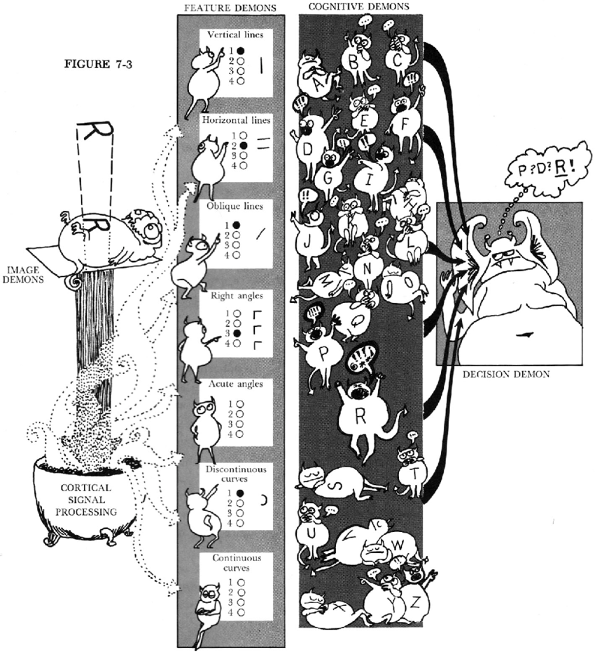
Oliver Selfridge’s “Pandemonium” (1959), a pattern learning system that learned to translate Morse code into text, is another creatural take on neural networks. Here, demons have replaced analogies of human vision. These creatures recognize and understand signals, identify with one particular letter and, when their assigned letter is called, shout into the ear of a decision demon.
Demons are supernatural beings that can be human or non-human, or spirits that have never inhabited a body. The disembodied demon may be a better stand-in for artificial intelligence than most other creatures, as its appearance shouldn’t be distracting.
Nonetheless, the figure of the demon is subject to the same problems of visualization as other concepts that we fail to imagine, except in our own image. It seems we lack the vocabulary and imagery to think and talk about intelligence without either invoking the animate or the spiritual.
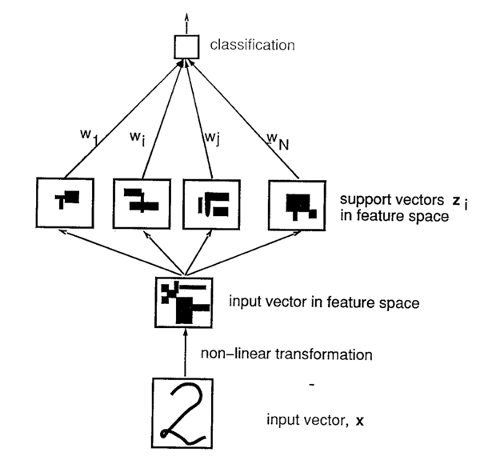
Over the decades, neural networks became increasingly complex. Multiple interconnected layers of neurons were introduced, allowing for more intricate computations and overcoming some of the restrictions of early neural nets. Better learning algorithms and increased computing power allowed for larger, deeper networks devouring ever-growing amounts of data.
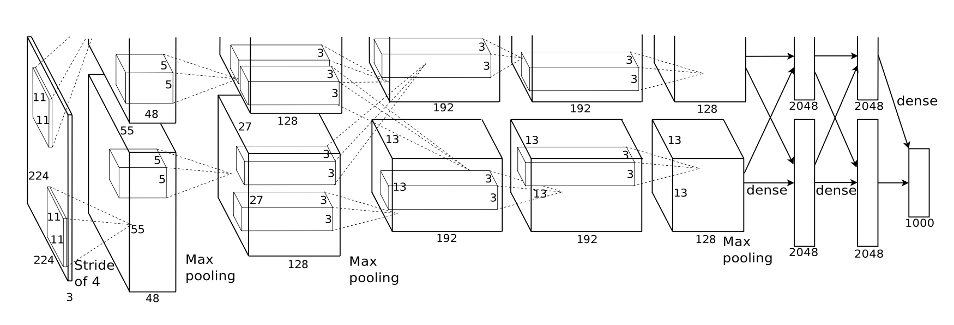
Modern deep learning neural networks are capable, in some cases, of doing things that humans do, and in a few cases even surpass our abilities. At the same time, the field has distanced itself from relating to human example and established itself as a separate, if contested, branch of intelligence.
We see this distancing reflected in diagrams: Modern neural network architectures are rendered in an increasingly abstract, flowchart-like diagrammatic similar to engineering. Earlier biomorphic connotations have disappeared from both the linguistic and visual vocabulary. The inaccuracy of the human stroke and the friction of pen on paper have succumbed to precisely generated computer graphics.
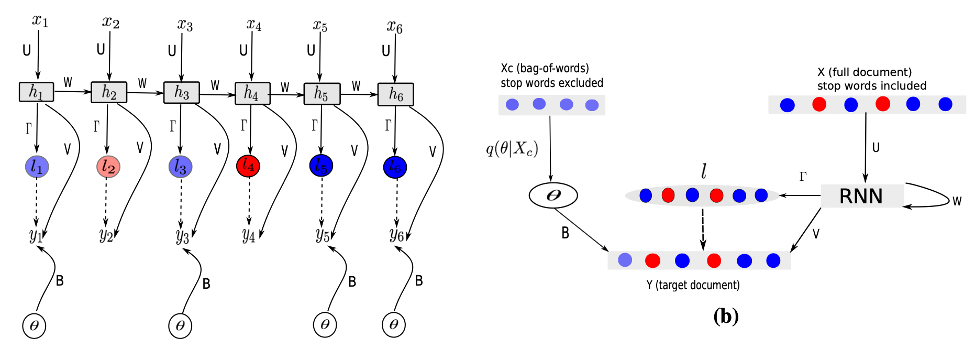
Neural networks are now painted as universally applicable, modular building blocks for learning and intelligent behavior, just as cyberneticians such as McCulloch and Pitts had imagined. Simultaneously, more and more researchers are including impact statements, model cards and nutrition labels in their publications to lend specificity to their algorithms. And AI explainability researchers are finding more and more ways to turn the mathematical processes of inhuman complexity into easy-to-understand images, descriptions and graphs.
So, do neural network diagrams make for a better portrait of AI than sci-fi tropes? Most people can’t understand them or relate to them like they can to a robot. But what if this is their best feature?
Instead of projecting onto a metal humanoid, a poetic reading of metaphor and symbolism in AI diagrams prompts us to consider how their creators think about cognition. This may lead to more productive conversations about the risks and opportunities of artificial intelligence research than we could have amid sci-fi tropes. And it elevates the question: What other conceptions of intelligence are desirable, and how can we represent them?