Reuben Cohn-Gordon is an AI researcher and writer. He is soon starting a postdoctoral position at the University of British Columbia in Bayesian inference and machine learning. Previously, he studied classics.
– ONE –
“He loves a whim without substance — what he thinks to be a body is a shadow.”
— Ovid, “Metamorphoses,” Book 3, line 417
The phrase “mere pattern recognition” tends to appear over and over again in discussions of modern advances of AI. What it gestures at is a perceived dichotomy between true understanding and a shallow surface-level trick that gives an interlocutor the impression they are talking to an agent that understands.
The apparent distinction is neatly embodied by the difference between an echo and Echo. An echo doesn’t understand what you said — you could scream in Amharic, Swahili or English, and the distortion produced by an echo would be much the same. Echo, on the other hand, is a person: a figure from Greek myths made famous in Ovid’s “Metamorphoses” who lurks in caves and repeats back what she hears. (The traditional story is that she does this because of a curse, but she prefers to think of it as an elaborate piece of performance art in the style of Oulipo.)
Echo understands what the sound you made means. When you say, “Where are you?” she apprehends more than just a series of vibrations in the air. She gets the information, the meaning, the content. “Where are you?” she replies, tauntingly, implying that you’re a busybody.
In an abstract sense, Echo and an echo are similar, both being machines that receive a sound and spit out a sound in return. But in another sense, they couldn’t be more different. One merely gives the impression of being a mordant interlocutor, the other actually is one.
As for a conversational AI system like the now terminally over-discussed ChatGPT, it too has the trappings of a real interlocutor, returning back words in answer to the words you give it.
Me: If computers were made of wood, would they work?
ChatGPT: No, computers need electricity and metal components to function.
Me: Why?
ChatGPT: Wood is an insulator, meaning it does not conduct electricity. Without electricity, a computer cannot function. Additionally, wood is not strong or durable enough to withstand the physical pressures of a computer’s components.
Me: What about a conductive hardwood?
ChatGPT: While some hardwoods may be slightly conductive, it would still not be enough to power a computer. The conductivity of the wood would be far too low to support the electrical components of a computer.
And so on. If deep learning systems from the 2010s onwards began to gesture toward semantic understanding, ChatGPT practically flaunts its flexible, fluent ability to reason counterfactually, smoothly taking into account both linguistic style and world knowledge.
But the perennial accusation against modern statistical AI, no matter how impressive it appears, is that it is less like Echo than her lowercase cousin, an operation on form when it should be an operation on content. Or like ELIZA, a program from the 1960s that rearranged what you said as a question, giving the impression of being uppercase Echo, not unlike the way two children stacked on top of each other in a trench coat gives the superficial impression of an adult. The meaning you perceive from ChatGPT’s responses, in this view, is only what you project onto it, a more elaborate version of perceiving a mocking tone in an echo.
The warning given by a host of like-minded linguists and cognitive scientists is that purely statistical approaches, being conceptually flawed, will hit a ceiling soon enough, with all the hubris of Icarus flying too near the sun. This will trigger an AI winter as the stock of OpenAI plummets into the chilly Adriatic.
That this criticism is so pervasive, despite the extraordinary behavior of modern AI systems, is a testament to an enduring analogy between the mind and software. As far as computer programming is concerned, the difference between echo and Echo, and the concomitant danger of confusing the two, is a familiar theme.
Programs are sequences of characters, while their meaning, or their content, are numbers or functions or other sorts of mathematically exact entities. One could imagine the forms of all programs living in one space (picture Borges’ infinite library from the short story “The Library of Babel,” if you are that way inclined), which contains every possible sequence of characters. One could also imagine the meanings of all possible programs living serenely in another space, one of mathematical functions.
The relationship between the two “libraries” is at the bedrock of computer science and mathematical logic, but it is equally relevant to everyday software writing tasks: Programs work by manipulating content, not form. For example, a chess program might take a string of characters like “8/8/8/4p1K1/2k1P3/8/8/8 b – – 0 1” as input (form) and extract from this the state of a chessboard (content). It might then perform some calculation on the basis of this content, like determining whether black can capture any pieces (yes/no).
This is a machine that takes form (string of characters) and produces form (yes/no), but trying to write this function without routing it through the content (the state of the chess board) and the surrounding concepts (the rules of chess) would be a doomed exercise.
In fact, a program that commits this sin has a name: It is a hacky program.

– TWO –
“Where do you flee to when I reach after you?”
— Ovid, “Metamorphoses,” Book 3, line 455
But what does this intuition about programming have to do with AI? One of the foundational premises of what is sometimes called classical AI is that human language is fundamentally similar to a programming language. In a programming language, the form is a series of letters or characters. Likewise in a human language, the form is a series of letters or characters: a sound.
In programming languages, the content — what the program does — is the world of numbers, functions and data, like the state of a chessboard. A fundamental idea of classical AI is that the “chessboard of the mind,” as it were, is the world, and concepts like politics and war, as well as specific historical events like the First World War, can be defined in terms of this chessboard.
Consider the sentence “If Archduke Franz Ferdinand had not been assassinated, the First World War would still have happened.” Assessing the truth of that sentence would be based on an understanding of the geopolitical situation at the beginning of the 20th century, a knowledge of how wars start, a consideration of how else the war could have started and so on. It would need to take into account possibilities like an agreement to defuse various tensions and judge this on the basis of the negotiating abilities of relevant leaders.
The chessboard around which this process of interpreting human language revolves is not exactly the world in the physical sense. The world that natural language is concerned with is filled with all sorts of things: objects like cats, stars or an awful pair of novelty socks; events like wars, birthdays or the act of wearing something you hate; sensations like dismay or the prickliness of wool against your ankles; and concepts like moral responsibility, beauty or passive aggressive gift-giving.
Wilfrid Sellars, a philosopher of language, termed this world of human things the “manifest image” to contrast it against the world of atoms bumping around in the void (the “scientific image”), which is better understood.
In short, the key idea of classical AI is to build a conceptual bridge from the mathematical world of computer science to the very human phenomenon of intelligence. On the left bank is the well-behaved world of mathematical form and content, and on right, the world of the manifest image. The bridge comes equipped with a friendly sign that says: Don’t worry, you can cross for free! That is to say, why not apply all the techniques of writing programming languages to understanding human ones, and all the techniques of writing software to understanding the mind.
It’s not hard to see why this perspective has been so pervasive. We constantly make use of the twin notions of form and content in our everyday interaction with the world. Take the four pictures in this article. Each is of Narcissus, another character from Ovid’s “Metamorphoses,” who stares longingly at what he believes is a stranger looking up at him from the surface of a pond. Each image differs entirely in form (the colors of the pixels in the respective images) but shares some abstract content.
“You feel as if classical AI proponents constantly want to scream: This is ridiculous! It’s all echo! None of this has anything to do with intelligence!”
For a linguistic example, consider that if you talk to someone about the Harry Potter books who has read them in French, you are not talking about the form (the sequence of letters that make up the book) but about some sort of content: the events that happen, the social institutions in the world, the characters, their intentions.
To happily accept that a book can be translated or that a painting can be done in a new style is to admit, albeit implicitly, some notion of content on the “right bank.”
It is this bridge that people have in mind when they talk about “true” understanding: minds extracting content from the blooming, buzzing confusion of sensory data, and scientists extracting the software of the mind from the evidence of behavior and biology. The vision of classical AI extends well beyond language and aims, in principle, to apply the tools of software to all kinds of things that people do, like buttering toast, designing cathedrals or politely excusing themselves from an awkward dinner party.
Statistical AI, on the other hand, exhibits none of the principles that the bridge implies. A system like ChatGPT embodies this strange fact perfectly: It may respond cogently to questions made up of long, linguistically complex sentences, but if you take apart the software, you won’t find a grammatical description of English anywhere. It might answer questions that require considered counterfactual reasoning or detailed knowledge about the world, but nowhere inside the machine will you find rules by which it reasons or a database of things that it knows.
This is hardly a surprise — no one put those things in. No programmer sat down and painstakingly explained the rules of English grammar to ChatGPT in its inchoate form, or patiently entered a history of the world into a database. Everything it knows, it knows only from the vast reams of text it has read.
It is also not surprising that a set of more classically minded AI researchers are reacting to ChatGPT, its contemporaries and its spiritual predecessors with distaste. For example, the cognitive scientist Douglas Hofstadter and political activist David Bender supplied the following questions to GPT-3 (a precursor to ChatGPT) for an article in The Economist, and they pointed to the failure of understanding implied by its nonsensical answers.
Dave & Doug: What do fried eggs (sunny side up) eat for breakfast?
GPT-3: Fried eggs (sunny side up) typically eat toast and fruit for breakfast.
Dave & Doug: How many parts will the Andromeda galaxy break into if a grain of salt is dropped on it?
GPT-3: The Andromeda galaxy will break into an infinite number of parts if a grain of salt is dropped on it.
Behaviors like this tend to trigger aha! moments from critics, as if the mask has been yanked off and the babbling meaningless brook of semantic white noise has been exposed. Failures to do consistent arithmetic fall in the same category: purported evidence that there is no one behind the wheel, no real understanding at work.
And when a successive system appears to do better, focus shifts to a new failing. ChatGPT answers the first question much more sensibly:
ChatGPT: Fried eggs, also known as sunny side up eggs, do not eat anything for breakfast because they are not a living organism. Fried eggs are a type of egg preparation in which eggs are cooked by being fried on one side with the yolk remaining runny.
And also the second:
ChatGPT: It is not possible to answer this question with any degree of accuracy, as it is based on a number of assumptions that are not grounded in reality. First, the Andromeda galaxy is a vast collection of stars, gas, dust, and other matter that is held together by its own gravity. It is not a solid object that can be broken into pieces, as you might break a piece of glass or ceramic by dropping something on it. Second, even if we assume that the Andromeda galaxy were a solid object that could be broken into pieces, the number of pieces into which it would break would depend on a number of factors, such as the size, shape, and composition of the galaxy, as well as the size, shape, and mass of the grain of salt.
Unsurprisingly, therefore, criticisms of ChatGPT tend to focus less on its ability to understand the world and answer previously unseen questions, and more on the huge amount of data needed for it to learn anything (apparently unlike people), its lack of social grounding and the factually inaccurate statements that it will produce with total confidence — as if it has learned, from the wealth of examples on the internet, to perform masculinity.
A final hold-out by traditionalists, if you really press the case, is that ChatGPT does do tasks like summary, essay writing or translation well, but this is evidence that the tasks were not interesting in the first place. The generation of beautiful art, remarkably clever poetry that satisfies the stylistic and semantic demands of a user and even accurate descriptions of images are all consigned to the wastebasket of uninteresting tricks by dint of being accomplished by the Wrong Kind of AI.
Like a friend with whom you keep having the same petty arguments, these icy reactions point to an underlying tension. You feel as if classical AI proponents constantly want to scream: This is ridiculous! It’s all echo! None of this has anything to do with intelligence! Some of them do scream that.
For them, it is like every absurd Borges story about philosophy rolled into one. It is like “The Library of Babel,” where scholars search for knowledge about the world not by exploring the space of ideas, but by literally trying to read every possible permutation of symbols. It is like “Pierre Menard, Author of the Quixote,” where the eponymous protagonist rewrites “Don Quixote” not by restating the plot in modern language, but word for word. And worst of all, it is like “Funes the Memorious,” a story about a man who remembers everything, but understands nothing.
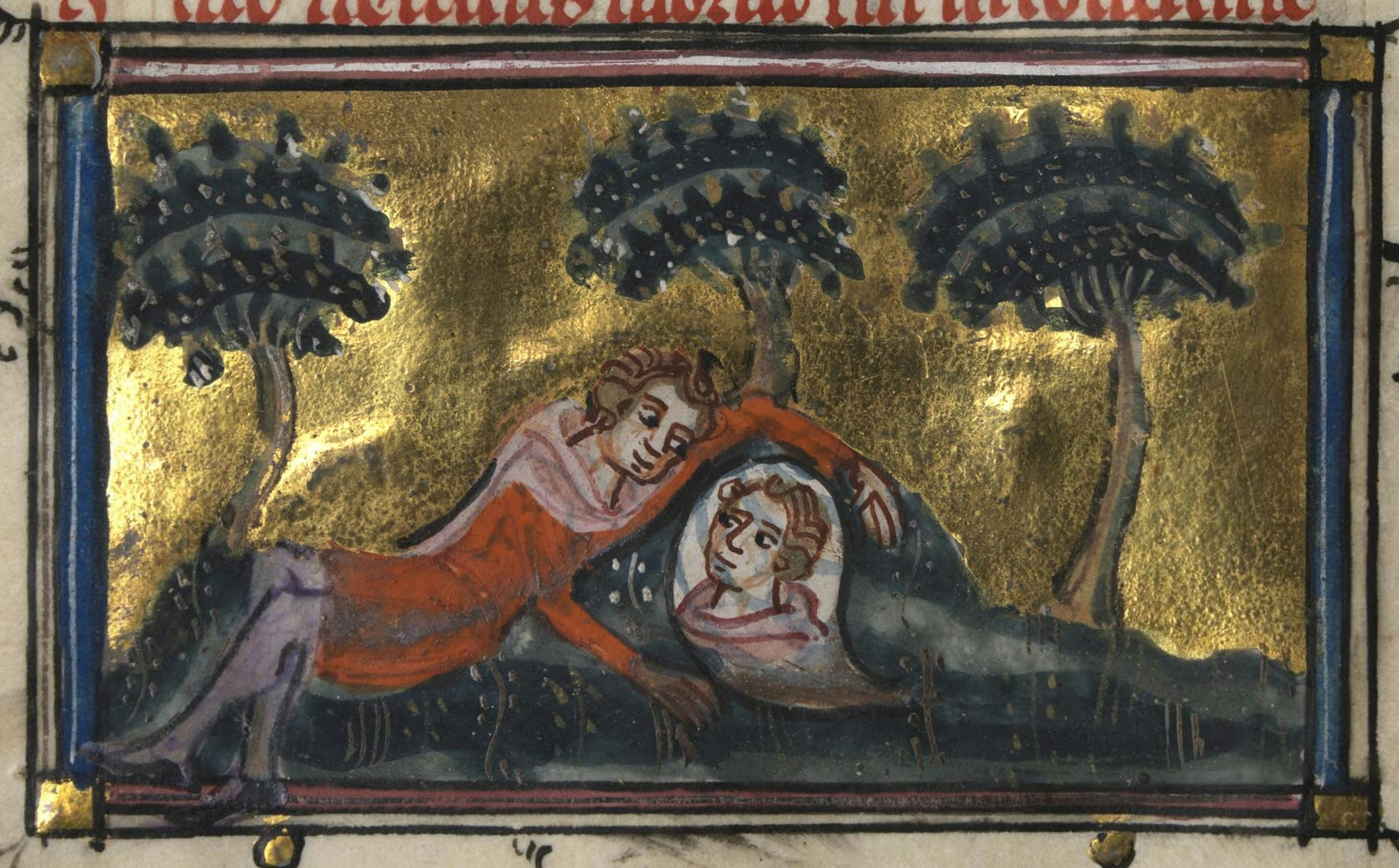
– THREE –
“Why, foolish one, do you seek an illusion in vain? What you seek is nowhere to be found.”
— Ovid, “Metamorphoses,” Book 3, line 432
But beyond all that, the deepest reason for the animosity is that modern statistical AI is like an old enemy returned from beyond the grave. Or really one of several, depending on the length of your engagement with the field.
Thinking of recent times, the debate about conversational AI systems might seem like a rehashing of the last decade of similar discussions about statistical methods for translation, image classification, image captioning and other visual and linguistic tasks. All of these were met with a now-familiar mixture of wild enthusiasm by some and predictions by others that their surface-level understanding was a red herring in the search for true understanding.
If you are older, the situation will remind you of connectionism, a perspective on AI and cognition that became popular in the 1980s and avoided hard-coded facts and linguistic grammars in favor of the implicit knowledge contained by neural networks able to perform cognitive tasks. At the time, critics of connectionism argued that the structured nature of thought meant that the project was doomed, or at best, would end up emulating the classical approach.
If you are older still, with the longevity of Noam Chomsky, an academic Methuselah who now and always is contemptuous and certain in his takedowns, you might remember Burrhus Skinner (could there be a better name for a villain?) and the specter of behaviorism.
Behaviorism avoids attempts to talk about beliefs, intentions, goals, desires, plans, events, mental representations of objects and so on in favor of examining behavior: the way that an agent takes sensory data as input and produces actions as outputs. It was a response to behaviorism, most famously associated with Chomsky’s review of Skinner’s book “Verbal Behavior,” that provoked the wave of cognitive science and classical AI from the 1950s onwards. This movement aimed to look not just at input and output of the brain — the biological hardware of interest to neuroscientists — but at the computational structure in between: the software.
Skinner was not a fan of this idea. In a reply to Chomsky decades later, he concludes with a fiery set of accusations against what had by then become a standard perspective on the mind: “I accuse cognitive scientists of misusing the metaphor of storage. The brain is not an encyclopaedia, library or museum. … I accuse cognitive scientists of relaxing standards of definition and logical thinking and releasing a flood of speculation characteristic of metaphysics, literature and daily intercourse, perhaps suitable enough for such purposes but inimical to science.”
Knowledge: a database of facts; language: a series of rules; meaning: logical propositions. All this is clumsy and excessive literalism to Skinner, without any scientific merit. In short, he thinks the bridge between the manifest image and the scientific image is not sturdy at all, and talk of mental software can only ever be a metaphor.
Indeed, anyone who has tried and failed to pin down something as simple as what a government or a fight or a game is in precise terms will know that things in the manifest image are strangely elusive, even those as seemingly concrete and exact as words or physical objects.
“Ryle likens the act of asking about the processes inside the mind to a tourist visiting Oxford or Cambridge and seeing all the various departments, and then asking a local where the university is.”
Skinner was not alone in his skepticism. To the philosopher Gilbert Ryle, the elusiveness of things in the manifest image is not a coincidence, it is a symptom of a deeper confusion that he termed the “dogma of the ghost in the machine.” He likens the act of asking about the processes inside the mind to a tourist visiting Oxford or Cambridge and seeing all the various departments, and then asking a local where the university is.
The university is the collection of departments and colleges, rather than a separate building, and so the tourist is searching for it in the wrong way. The tourist, Ryle said, made a category mistake.
The archetype of the error comes from biologists in the 18th and 19th centuries who made the analogous mistake of searching for a life force (élan vital) that exists in addition to and separate from the physical body. They rejected any theory in which a person was a “mere” material object.
Ryle’s conviction is that people have a tendency to repeat this mistake in many forms, not least by searching for an essential ingredient of intelligence in addition to, and separate from, the act of intelligent behavior.
Indeed, as large language models produce increasingly obvious demonstrations of intelligence, the protestations that they are lacking a key ingredient become louder too: variously, the ability to be original, to be explainable, to be reliable and most of all, to speak meaningfully. Just look at the titles of a series of vexingly similar thought-pieces in well-known venues: “ChatGPT Is Dumber Than You Think” (The Atlantic); “The False Promise of ChatGPT” (The New York Times); “The stupidity of AI” (The Guardian).
In particular, the distinction between form and content — and the purported impossibility of statistical AI to grasp the latter — is the perfect essential property for critics to latch onto. It’s a crisp distinction between true understanding and a mere trick, but not one Ryle would believe in any more than the “élan vital” or any other diehard commitment to the dogma of the ghost in the machine.
If “be Echo, not echo” is the catchphrase of the classical AI perspective, then Narcissus staring at his reflection in a pond, trying to work out the nature of his unrequited beloved, is the skeptics’ motif for the search for “true understanding.”

– FOUR –
“It’s me! I understand and my own image fools me no more.”
— Ovid, “Metamorphoses,” Book 3, line 463
What would Ryle or Skinner make of a system like ChatGPT — and the claim that it is mere pattern recognition, with no true understanding?
The philosopher Daniel Dennett, a former student of Ryle’s, has spent his career developing “thinking tools” to expose what he sees as false dichotomies like this one. True free will versus mere freedom from constraint, true agents versus mere machines, true understanding versus mere pattern recognition.
A fan of making up whimsical names for useful ideas, he coined the handy term “deepity”:
A deepity is a proposition that seems both important and true and profound but that achieves this effect by being ambiguous. On one reading it is manifestly false, but it would be earth-shaking if it were true; on the other reading it is true but trivial. The unwary listener picks up the glimmer of truth from the second reading, and the devastating importance from the first reading, and thinks, Wow!
A classic example is the proposition that “a person is a pile of atoms.” The true but uninteresting reading is that a person is made up of atoms, and the interesting but false reading is that a person is simple and predictable because of it. The deepity performs a sleight of hand by wavering between the former and the latter.
Once armed with the idea of a deepity, you see them everywhere in the discourse about modern AI. There is the claim that “large language models are mere stochastic parrots,” a sublime example of a deepity. The true but uninteresting meaning is that large language models (like ChatGPT) produce words as a (probabilistic) function of previous words and other data. Well of course! You could say the same about a person, just like you could describe them as a collection of atoms.
The other meaning is the one that suggests that ChatGPT has no understanding of communicative intent, so when you ask it a question, it can only respond correctly in limited cases where it has seen the question, or else give awkward ill-fitting answers. But in this sense, ChatGPT is obviously not a stochastic parrot. You can ask it all sorts of subtle things, questions it has never seen before and which cannot be answered without understanding. For example:
Me: Translate the following sentence into the language spoken in the country of which Madrid is the capital: “I want to travel the world.”
ChatGPT: “Quiero viajar por el mundo.” This sentence is in Spanish, which is the official language of Spain, the country of which Madrid is the capital. The translation of “I want to travel the world” into Spanish is “Quiero viajar por el mundo.”
But does it understand communicative intent? Let’s probe further.
Me: What might be the communicative intent of saying this?
ChatGPT: The communicative intent of saying “I want to travel the world” might be to express a desire or ambition to see and experience different places and cultures around the globe. The speaker might be expressing a longing for adventure, a curiosity about the world, or a desire to broaden their horizons and learn new things. Alternatively, the speaker might simply be stating a personal preference or expressing a plan to travel extensively in the future. The specific communicative intent of the statement would depend on the context in which it is made and the relationships between the speaker and the listener(s).
Me: Give two different contexts, with different communicative intents, where one might say this.
ChatGPT: Here are two different contexts in which one might say “I want to travel the world,” with different communicative intents:
Context 1:
Speaker: A young person who is planning their gap year after high school graduation
Listener: A group of friends or family members who are discussing travel plans or future goals
Communicative intent: The speaker is expressing a desire to see and experience different parts of the world and is possibly seeking encouragement or support from the listener(s) in realizing this goal.
Context 2:
Speaker: A business owner who is seeking to expand their company’s global reach
Listener: A potential client or business partner
Communicative intent: The speaker is expressing their ambition to grow their business and reach new markets, and is possibly seeking to establish credibility or build rapport with the listener(s) by demonstrating a global perspective and willingness to take risks.
Having had an interaction like this, a new user of ChatGPT might feel rightly misled by the epithet “stochastic parrot” and the claim it is making about what ChatGPT can and cannot do.
Other deepities are less facile and more subtly misleading, like Ted Chiang’s claim that ChatGPT is “a blurry JPEG of the web.” In the unremarkable sense that any understanding is a compression or distillation of data, then sure. In the more interesting sense that ChatGPT cannot produce truly creative language, then of course not.
In a recent New York Times article, Chomsky and coauthors described ChatGPT as “a lumbering statistical engine for pattern matching, gorging on hundreds of terabytes of data and extrapolating the most likely conversational response or most probable answer to a scientific question.” This stylish description sounds profound, but as soon as it is converted into concrete predictions about what ChatGPT can or cannot do (quickly adapt to new instructions and data, give creative answers to previously unseen questions, explain its reasoning) it holds no water.
For better or worse, the urge to produce an insightful pronouncement about modern AI, and the temptation of a deflationary narrative in which the apparent miracle is shown to be a clever trick, has led to a cottage industry of opinions in this vein espousing similar deepities. Phrases like “a convincing bullshit generator,” “a glorified autocomplete” and “a surface level tool” abound.
And more tellingly, even people genuinely impressed by modern AI buy into this perspective. As the philosopher David Papineau wrote on Twitter: “Is ChatGPT dumb? Damn right it is. It’s just a next-word predictor, trained by trial and error to produce a likely next word given some text, and then a likely next word after that. … Myself, I’m astounded this simple process is enough to yield such elegant and germane speech.”
“ChatGPT is obviously not a stochastic parrot.”
The question is, why is it astounding? One senses that people’s expectations about what “mere” next-word prediction can do is limited by their imagination, or their implicit conflation of next-word prediction with the clumsy abilities of text autocomplete.
In that vein, Dennett likes to give the example of biologist William Bateson, who, before the discovery of DNA, rejected the possibility of a physical information-carrying mechanism in living things on the grounds of implausibility: “It is inconceivable that particles of chromatin or of any other substance, however complex, can possess those powers which must be assigned to our factors or genes. The supposition that particles of chromatin, indistinguishable from each other and indeed almost homogeneous under any known test, can by their material nature confer all the properties of life surpasses the range of even the most convinced materialism.”
Bateson could not imagine DNA, and many people cannot imagine that a mechanical system that has learned statistical correlations can think, have intentions or understand concepts.
And yet the fact is that it is remarkably easy to treat ChatGPT as an agent with beliefs, knowledge and understanding — or, to borrow another coinage from Dennett, to take the intentional stance towards it. The ease with which we can do this, and the degree to which the system’s behavior does not disabuse us of this stance, is startlingly greater than systems even just a few years old, and goes some way to explain the hype ChatGPT generated toward the end of 2022.
It is able not just to respond to questions but to respond in the way you’d expect if it did indeed understand what was being asked. And, to take the viewpoint of Ryle, it genuinely does understand — perhaps not as adroitly as a person, but with exactly the kind of true intelligence we attribute to one.
As is the cyclical way of these things, classical AI now finds itself losing ground to systems that evoke exactly the kinds of behaviorist perspectives it sought out to dismiss. If Ryle and Skinner were able to witness these developments, they would be doing whatever is the opposite of rolling in their respective graves. ChatGPT’s success is about the closest possible thing to a vindication of Skinner’s behaviorism and Ryle’s rejection of the ghost in the machine.
Narcissus eventually realizes that his elusive interlocutor is not a person lying underneath the surface of the pond, mirroring his every move, but his own reflection. At this point he throws a huge tantrum and turns into a flower.
But how does the analogous motif play out in AI? Will there be a philosophical sea-change, in which the distinction between true understanding and mere pattern recognition gives way to something new? Or, with Skinner and Ryle in mind, something old?