Shannon Vallor is the Baillie Gifford Chair in the Ethics of Data and Artificial Intelligence and the director of the Centre for Technomoral Futures at the University of Edinburgh. Her latest book is “The AI Mirror: How to Reclaim Our Humanity in an Age of Machine Thinking” (Oxford University Press).
It is a profoundly erroneous truism … that we should cultivate the habit of thinking of what we are doing. The precise opposite is the case.
Civilization advances by extending the number of important operations which we can perform without thinking about them.
— Alfred North Whitehead, “An Introduction to Mathematics,” 1911
GPT-3 is the latest attempt by OpenAI, a tech research lab in San Francisco, to unlock artificial intelligence with an anvil rather than a hairpin. As brute force strategies go, the results are impressive. The language-generating model performs well across a striking range of contexts. Given only simple prompts, GPT-3 writes not just interesting short stories and clever songs, but also executable code such as web graphics.
GPT-3’s ability to dazzle with prose and poetry that appears entirely natural, even erudite or lyrical, is less surprising. It’s a parlor trick that its predecessor performed a year earlier, though its then-massive 1.5 billion parameters are swamped by GPT-3’s power, which uses 175 billion parameters to enhance its stylistic abstractions and semantic associations.
Just like their great-grandmother, Joseph Weizenbaum’s ELIZA, a natural language processing program developed in the 1960s, these systems benefit considerably from human reliance on familiar heuristics for speakers’ cognitive abilities. GPT-3 readily deploys artful and sonorous speech rhythms, sophisticated vocabularies and references, and erudite grammatical constructions. Like the bullshitter who gets past their first interview by regurgitating impressive-sounding phrases from the memoir of the company’s CEO, GPT-3 spits out some pretty good bullshit.
Yet the connections GPT-3 makes are not illusory or concocted from thin air. It and many other machine learning models for natural language processing and generating do, in fact, track and reproduce real features of the symbolic order in which humans express thought. And yet, they do so without needing to have any thoughts to express.
The hype around GPT-3 as a path to general artificial intelligence reveals the sterility of mainstream thinking about AI today. More importantly, it reveals the sterility of our current thinking about thinking.
“Like the bullshitter who gets past their first interview by regurgitating impressive-sounding phrases from the memoir of the company’s CEO, GPT-3 spits out some pretty good bullshit.”
A growing number of today’s cognitive scientists, neuroscientists and philosophers are aggressively pursuing well-funded research projects devoted to revealing the underlying causal mechanisms of thought, and how they might be detected, simulated or even replicated by machines. But the purpose of thought — what thought is good for — is a question widely neglected today, or else taken to have trivial, self-evident answers. Yet the answers are neither unimportant, nor obvious.
The neglect of this question leaves uncertain the place for thought in a future where unthinking intelligence is no longer an oxymoron, but soon to be a ubiquitous mode of machine presence, one that will be embodied in the descendants and cousins of GPT-3. There is an urgent question haunting us, an echo from Alfred North Whitehead’s conclusion in 1911 that civilizations advance by expanding our capacity for not thinking: What thoughts do the civilized keep?
Whitehead, of course, was explicitly talking about the operations of mathematics and the novel techniques that enable ever more advanced shortcuts to be taken in solving mathematical problems. To suggest that he is simply wrong, that all operations of thought must be forever retained in our cognitive labors, is to ignore the way in which shedding elementary burdens of thought often enables us to take up new and more sophisticated ones. As someone who lived through the late 20th century moral panic over students using handheld scientific calculators in schools, I embrace rather than deny the vital role that unthinking machines have historically played in enabling humans to stretch the limits of our native cognitive capacities.
Yet Whitehead’s observation leaves us to ask: What purpose, then, does thinking hold for us other than to be continually surpassed by mindless technique and left behind? What happens when our unthinking machines can carry out even those scientific operations of thought that mathematical tables, scientific calculators and early supercomputers previously freed us to pursue, such as novel hypothesis generation and testing? What happens when the achievements of unthinking machines move outward from the scientific and manufacturing realms, as they already have, to bring mindless intelligence further into the heart of social policymaking, political discourse and cultural and artistic production? In which domains of human existence will thinking — slow, fallible, fraught with tension, uncertainty and inconsistency — still hold its place? And why should we want it to?
The Labor Of Understanding
To answer these questions, we need to focus on what unthinking intelligence lacks. What is missing from GPT-3?
It’s more than just sentience, the ability to feel and experience joy or suffering. And it’s more than conscious self-awareness, the ability to monitor and report upon one’s own cognitive and embodied states. It’s more than free will, too — the ability to direct or alter those states without external compulsion.
Of course, GPT-3 lacks all of these capacities that we associate with minds. But there is a further capacity it lacks, one that may hold the answer to the question we are asking. GPT-3 lacks understanding.
This is a matter of some debate among artificial intelligence researchers. Some define understanding simply as behavioral problem-solving competence in a particular environment. But this is to mistake the effect for the cause, to reduce understanding to just one of the practical powers that flows from it.
For AI researchers to move past the behaviorist conflation of thought and action, the field needs to drink again from the philosophical waters that fed much AI research in the late 20th century, when the field was theoretically rich, albeit technically floundering. Hubert Dreyfus’s 1972 ruminations in “What Computers Can’t Do”(and 20 years later, in “What Computers Still Can’t Do”) still offer many soft targets for legitimate criticism, but his and other work of the era at least took AI’s hard problems seriously. Dreyfus in particular understood that AI’s true hurdle is not performance but understanding.
Understanding is beyond GPT-3’s reach because understanding cannot occur in an isolated computation or behavior, no matter how clever. Understanding is not an act but a labor. Labor is entirely irrelevant to a computational model that has no history or trajectory in the world. GPT-3 endlessly simulates meaning anew from a pool of data untethered to its previous efforts. This is the very power that enables GPT-3’s versatility; each task is a self-contained leap, like someone who reaches the flanks of Mt. Everest by being flung there by a catapult.
“Understanding is beyond GPT-3’s reach because understanding cannot occur in an isolated computation or behavior, no matter how clever.”
GPT-3 cannot think, and because of this, it cannot understand. Nothing under its hood is built to do it. The gap is not in silicon or rare metals, but in the nature of its activity.
Understanding does more than allow an intelligent agent to skillfully surf, from moment to moment, the associative connections that hold a world of physical, social and moral meaning together. Understanding tells the agent how to weld new connections that will hold under the weight of the intentions, values and social goals behind our behavior.
Predictive and generative models like GPT-3 cannot accomplish this. GPT-3 doesn’t even know that to successfully answer the question “Can AI be conscious?,” as the philosopher Raphaël Millière prompted it to do in an essay, it can’t randomly reverse its position every few sentences.
GPT-3 effortlessly completed the essay assigned by Millière. This is a sign not of GPT-3’s understanding, but the absence of it. To write it, it did not need to think; it did not need to struggle to weld together, piece by piece, a singular position that would hold steady under the pressure of its other ideas and experiences, or questions from other members of its lived world.
The instantaneous improvisation of its essay wasn’t anchored to a world at all; instead, it was anchored to a data-driven abstraction of an isolated behavior-type, one that could be synthesized from a corpus of training data that includes millions of human essays, many of which happen to mention consciousness. GPT-3 generated an instant variation on those patterns and, by doing so, imitated the behavior-type “writing an essay about AI consciousness.”
But it did not need to know anything about what an essay on AI consciousness might seek to do, or how it would fit into the larger world of social meaning that makes the subject of AI consciousness worth seeking to understand. It is akin to the difference between a songbird’s tuneful mimicry of a human lullaby and a new human father’s variation — however tuneless — on the lullaby his mother once sang to him as a child. One act is anchored in an understanding of the shared social history of meaning that gives a lullaby significance. The other is not.
Understanding is a lifelong labor. It is also one carried out not by isolated individuals but by social beings who perform this cultural labor together and share its fruits. The labor of understanding is a sustained, social project, one that we pursue daily as we build, repair and strengthen the ever-shifting bonds of sense that anchor our thoughts to the countless beings, things, times and places that constitute a world. It is this labor that thinking belongs to.
When GPT-3 is unable to preserve the order of causes and effects in telling a story about a broken window, when it produces laughable contradictions within its own professions of sincere and studied belief in an essay on consciousness, when it is unable to distinguish between reliable scholarship and racist fantasies — GPT-3 is not exposing a limit in its labor of understanding. It is exposing its inability to take part in that labor altogether.
Thus, when we talk about intelligent machines powered by models such as GPT-3, we are using a reduced notion of intelligence, one that cuts out a core element of what we share with other beings. This is not a romantic or anthropocentric bias, or “moving the goalposts” of intelligence. Understanding, as joint world-building and world-maintaining through the architecture of thought, is a basic, functional component of human intelligence. This labor does something, without which our intelligence fails, in precisely the ways that GPT-3 fails.
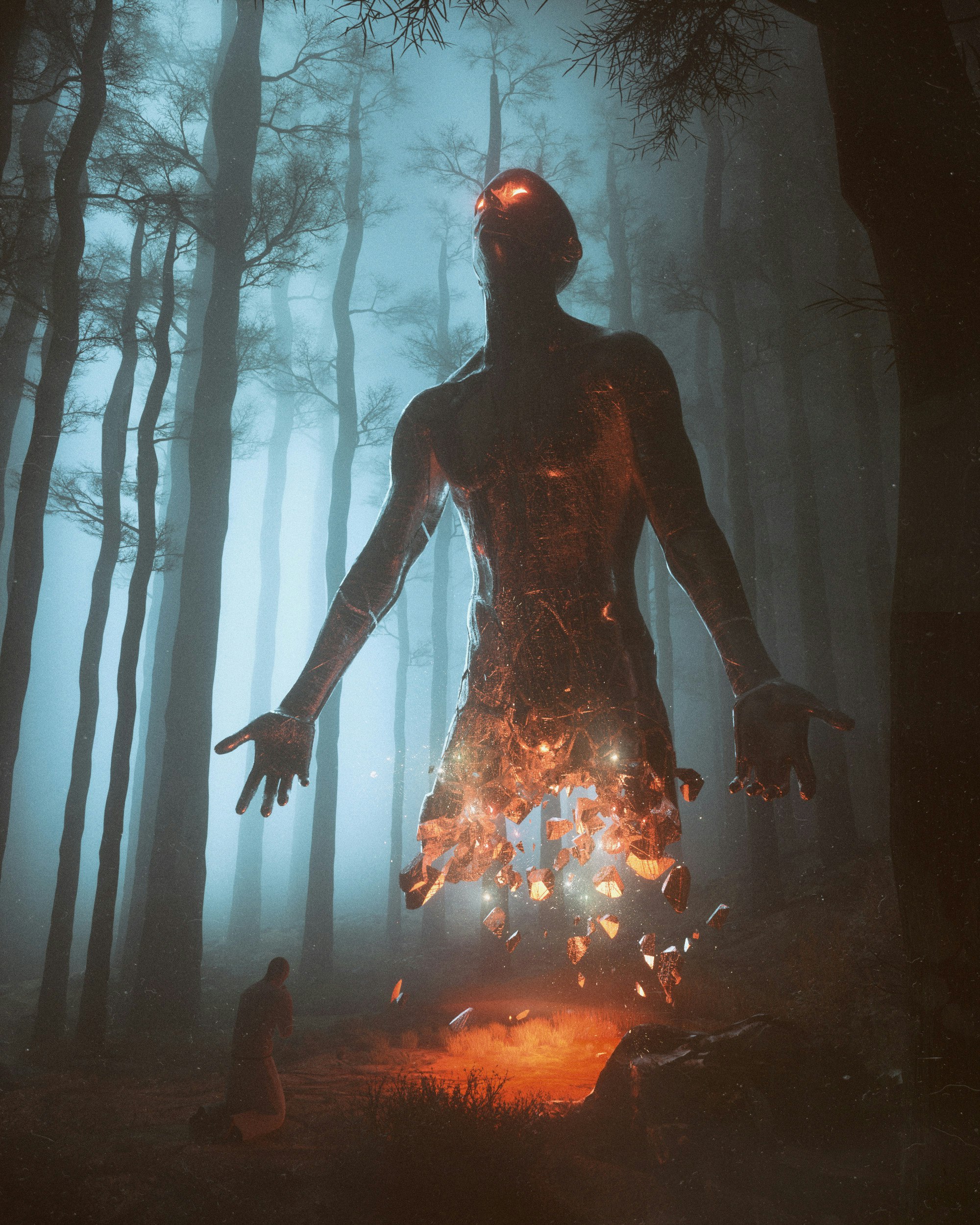
A Legacy In Danger
While machines remain wholly incapable of the labor of understanding, there is a related phenomenon in the human world. Extremist communities, especially in the social media era, bear a disturbing resemblance to what you might expect from a conversation held among similarly trained GPT-3s. A growing tide of cognitive distortion, rote repetition, incoherence and inability to parse facts and fantasies within the thoughts expressed in the extremist online landscape signals a dangerous contraction of understanding, one that leaves its users increasingly unable to explore, share and build an understanding of the real world with anyone outside of their online haven.
Thus, the problem of unthinking is not uniquely a machine issue; it is something to which humans are, and always have been, vulnerable. Hence the long-recognized need for techniques and public institutions of education, cultural production and democratic practice that can facilitate and support the shared labor of understanding to which thought contributes. Had more nations invested in and protected such institutions in the 21st century, rather than defunding and devaluing them in the name of public austerity and private profit, we might have reached a point by now where humanity’s rich and diverse legacies of shared understanding were secured around the globe, standing only to be further strengthened by our technological innovations.
Instead, systems like GPT-3 now threaten to further obscure the value of understanding and thinking. For as their narrow competence and frequently unreliable performance is gradually supplanted by more stable, adaptable and robust forms of unthinking intelligence, AI systems will appear to be a far more attractive source of prudent decision-making and governance. This will certainly be true if the primary alternative is reliance upon an increasingly disordered tumult of conspiracy-addled humans struggling to hold together even the shared fruits of understanding that prior generations produced.
And so, if the breathlessly over-hyped warnings from Elon Musk, Bill Gates and others of artificially intelligent machines “taking over” our human affairs have any grounding in reality, it comes not from the imminent rise of machines that understand more than we do, but from our collective and institutional failures to preserve our own capacities for this labor.
“Extremist communities, especially in the social media era, bear a disturbing resemblance to what you might expect from a conversation held among similarly trained GPT-3s.”
In an era where the sense-making labor of understanding is supplanted as a measure of human intelligence by the ability to create an app that reinvents another thing that already exists — where we act more like GPT-3 every day — it isn’t a surprise that GPT-3 might be mistaken for the AI breakthrough that will spawn true machine intelligence. But as AI researcher Gary Marcus and many others have acknowledged, that goal awaits in a different direction. If machines ever do join us in the domain of understanding — if they become able to think, know and build new worlds with us or with one another — then GPT-3 will be a footnote in their story.
But even as someone who thinks about AI for a living, I don’t find myself worrying much about when, or if, machines will get there. I find myself worrying about whether, by the time they do, we will still be capable of thinking and understanding alongside them. We can get by and endure, for a while, by riding the coattails of those who labored before us. But not for much longer, unless we repair and restart the engines of thinking for future generations — the cultural institutions, social practices, norms and virtues that valorize and enable, rather than penalize and suppress, the shared human labor of understanding.
Humanity has reached a stage of civilization in which we can build space stations, decode our genes, split or fuse atoms and speak nearly instantaneously with others around the globe. Our powers to create and distribute vaccines against deadly pandemics, to build sustainable systems of agriculture, to develop cleaner forms of energy, to avert needless wars, to maintain the rule of law and justice and to secure universal human rights — these are the keys to our future.
Yet they are all legacies of past labors of understanding that even now we wield with increasingly unsteady and unthinking hands. Of course, these achievements would all be impossible if Whitehead’s words were not in large part true. But we have failed to seriously ask the question that should have followed: “What thoughts do the civilized keep?”